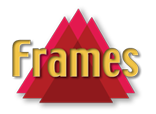
Resource Catalog
Document
The technologies and models based on machine vision are widely used for early wildfire detection. Due to the broadness of wild scene and the occlusion of the vegetation, smoke is more easily detected than flame. However, the shapes of the smoke blown by the wind change constantly and the smoke colors from different combustors vary greatly. Therefore, the existing target detection networks have limitations in detecting wildland fire smoke, such as low detection accuracy and high false alarm rate. This paper designs the attention model Recursive Bidirectional Feature Pyramid Network (RBiFPN for short) for the fusion and enhancement of smoke features. We introduce RBiFPN into the backbone network of YOLOV5 frame to better distinguish the subtle difference between clouds and smoke. In addition, we replace the classification head of YOLOV5 with Swin Transformer, which helps to change the receptive fields of the network with the size of smoke regions and enhance the capability of modeling local features and global features. We tested the proposed model on the dataset containing a large number of interference objects such as clouds and fog. The experimental results show that our model can detect wildfire smoke with a higher performance than the state-of-the-art methods.
Cataloging Information
- BiFPN - bidirectional feature pyramid network
- feature enhancement
- smoke detection
- Swin Transformer
- wildfire
- wildland fire