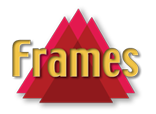
Resource Catalog
Document
In 2021, the availability of a physical model for lightning density prediction at ECMWF led the development of data driven models to identify episodes conducive of fires. The machine-learning classifiers worked remarkably well reaching an overall accuracy up to 78%. Still, errors in the lightning forecast substantially reduce the prediction skill. An ensemble of predictions is used to take into account the stochastic nature of the forecast errors. An assessment of the predictive skill in terms of probabilities is performed and is bench-marked against the deterministic outcome provided by a single deterministic realisation. The probabilistic prediction is more skilful than the deterministic one and there is an optimal probability threshold that allows to maximise the correlation between prediction and observed ignitions. Probabilistic forecast also brings the best value for money when a cost-loss analysis is performed, and a potential economic value is attributed to the forecast.
Cataloging Information
- fire prediction
- lightning ignited fires